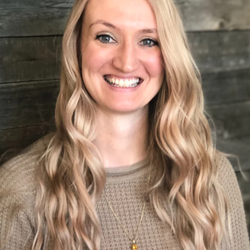
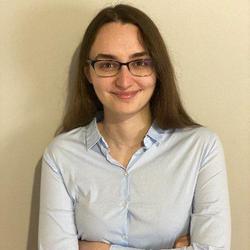
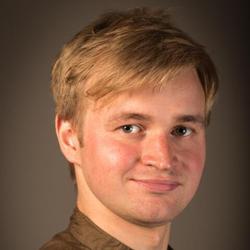
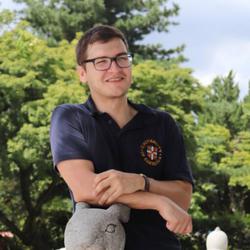
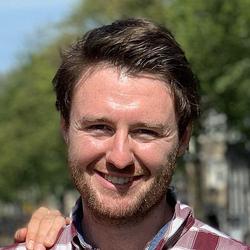
Graph Machine Learning - Research and industry applications
A Talk by Andreea Deac , Mikhail Galkin , Dr. Charles Hoyt , Dr. Tiffany Callahan and Sergei Ivanov
About this Talk
Description
Graph-based technologies became first-class citizens in various industries and many practical applications. Still, building performant and reliable machine learning pipelines over graph data, e.g., graph machine learning applications and products, remains a non-trivial task.
This panel discussion brings together academic and industrial experts from fields where Graph ML yields significant gains and greatly improves traditional processes. In addition to highlighting successful business cases, the panel concentrates on questions often dismissed or hidden behind the curtains of modern Graph ML applications.
In particular, we will talk about the origins of graph data, its modeling, organization, and processing aspects; best communication interfaces; bridging a gap between products and ML algorithms as well as measuring their practical impact. On a higher level, the panel will discuss upcoming trends in industrial Graph ML and prospective disruptive applications.
Key Topics
- Graph Machine Learning
- Deep Learning
- Graph Data Management
- Knowledge Graphs
- Graph ML in Production
Target Audience
- Machine Learning Practitioners
- Data Scientists
- Data Modelers
- CxOs
- Investors
Goals
- Explore the interplay between machine learning and knowledge based technologies
- How to get the “actionable” knowledge from the graph data?
- How can those approaches complement one another, and what would that unlock?
- What is the current state of the art, how and where is it used in the wild?
- NLP
- Biomed
- Industry (say, Ad Tech)
- What are the next milestones / roadblocks?
- Where are the opportunities for investment?
- We know drug discovery is on its highs, NLP is being democratized rapidly, what else?
Session outline:
- Introduction
- Meet and Greet
- Setting the stage
- Knowledge Graphs, meet Graph Machine Learning
- It’s all about the data:
- How do you create, maintain, and process graphs?
- Databases or tabular sources?
- Do you consider data modeling aspects?
- It’s all about the data:
- Best communication interface: natural language or structured query languages?
- How can machine learning help create and populate graphs (including KGs)?
- Cover some of the current state of the art
- An edge - does it appear naturally or derived from node similarities?
- What kind of problems can we solve by using it?
- NLP, Biomed, Industry (say, adtech)
- How academic datasets align with real-world tasks
- Where is this used in production?
- Success stories and business cases
- What are the major roadblocks / goals, how could we address them, and what would that enable?
- How to bridge the gap between business goals and Graph ML models?
- How do we measure the impact of applying ML models in real-world tasks? Metrics, A/B testing, generally about setting things in production
- Who are some key players to keep an eye on?
- Both from industry and research
Format
- Extended panel
- Expert discussion, coordinated by moderator
- 2 hours running time
- Running time includes modules of expert discussion, interspersed with modules of audience Q&A / interaction
Level
Intermediate - Advanced
Prerequisite Knowledge
- Basic understanding of Knowledge Graphs
- Basic understanding of Machine Learning / Deep Learning
You need an access pass to attend this session: Diversity Access Pass or Full Access Pass apply