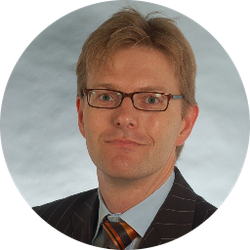
How a Knowledge Graph Can Support Advanced Price Analytics in Supply Chain Management
A Talk by Marcus Nölke (Cost Value Engineer & Data Analyst, Siemens Energy)
About this Talk
In a company with high purchasing volume, it is crucial to design cost-efficient parts and procure these parts at competitive pricing level.
Data analytics can support on both aspects. Unfortunately, supply chain transactional data but also product life cycle data can be messy and distributed among many applications and data silos.
For the task to determine if a particular offer or paid price for a part is reasonable, the following two problems need to be solved:
Definition of scope: What do I get for the price? Are there any options included? Is packaging and transportation part of the offer?
Definition of requirements/features: What is the underlying product specification of this offer? What is the material? What special requirements does it fulfill?
Therefore, pricing information needs to be connected to design information and a clear designation system for scope and requirements must be developed in a way that it can be formally represented by a machine.
This presentation will provide insight on how a Knowledge Graph is used to deal with heterogenous data input. Additionally, an example will show how automated pricing analytics is possible using semantics and logic available in the Knowledge Graph.
A Knowledge Graph can serve here as a data integration layer based on a shared vocabulary, defined relations and properties for parts and components of which the company’s products are made of.
A crucial role plays the use of core ontologies applicable for industrial and financial domain such as the ISO 15926-14 or the FIBO ontologies. QUDT is helpful in providing a solid basis for units and measurements.
Additional domain ontologies e.g. for purchasing or engineering help to include semantics and relations among the specific domain data elements.
The transactional purchasing data as well as product design data will be mapped to the concepts and relations defined in the core and domain ontologies.
With the help of a reasoning engine automatic identification, classification and relation mapping is done. This results in automated pricing evaluations possible for most parts of the purchasing volume.
For instance, the inlet system for a gas turbine is modeled with properties such as material or inlet mass flow. Past and current pricing of the inlet system is available from the global purchasing organization for the entire gas turbine portfolio ranging from small engines to very large engines.
Using the above inlet system data model with the defined properties a regression chart can be established showing inlet system pricing over mass flow and material categories. Both properties are known to be the major cost drivers for such systems.
The regression chart can be used to determine an average price level or for detecting price anomalies. E.g. an specific offer can be evaluated against the average or even lowest price line.
This procedure is also called (multi) linear performance pricing (see https://commons.wikimedia.org/wiki/File:Linear_Performance_Pricing.png) where sales prices are shown related to performance. Performance is an aggregated value considering the important part properties.
Using a Knowledge Graph with reasoning capabilities it is possible to apply linear performance pricing at scale for all parts which need to be purchased within a company. The relevant data can be automatically mapped from legacy data bases into the Knowledge Graph. No manual data collection, curation and update processes are necessary.
Talk+Live Q&A at the Western Auditorium in Connected Data World Center
You need an access pass to attend this session: Diversity Access Pass or Full Access Pass apply